Notes: RETHINKING ARCHITECTURE SELECTION IN DIFFERENTIABLE NAS
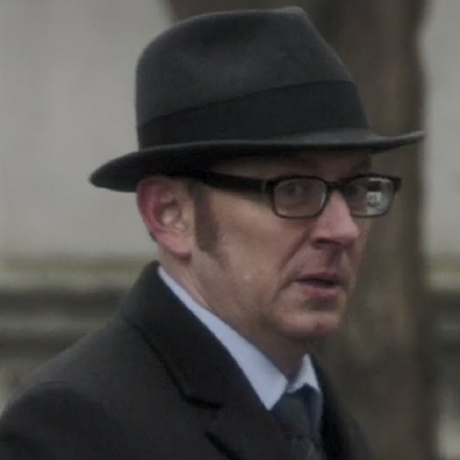
简介
提出DARTS中的
方法
在darts的supernet训练好之后,进行perturbation-based architecture selection
(PT),包括两点:
- 去掉一个operator,用accuracy下降的大小来作为operator选择的依据
- 逐步离散化edge,每确定一个edge上的operator就fintune一次
具体如下
实验与结论
进行了如下实验:
- Comparison with state-of-the-art image classifiers on CIFAR-10. 在现有的DARTS类方法上加上了PT,分数都得到了提升。
- Robustness issue of DARTS can be explained by the failure of
magnitude-based architecture selection. 实验验证了DARTS在某些搜索空间中失效的现象可以通过PT避免 - test a baseline by combining progressive tuning with magnitude-based operation selection instead of our selection criterion。证明论文提出的评价指标确实更好体现了operator的strength。
- 实验证明了在supernet的训练过程中
可以固定为0(即去掉 ),使用PT后性能没有下降
- Post title:Notes: RETHINKING ARCHITECTURE SELECTION IN DIFFERENTIABLE NAS
- Post author:shaw
- Create time:2021-09-14 21:15:53
- Post link:https://www.zenwill.top/2021/09/14/Rethinking gradient-based nas/
- Copyright Notice:All articles in this blog are licensed under BY-NC-SA unless stating additionally.
Comments